Understanding Malware Machine Learning: A Game Changer in IT Security
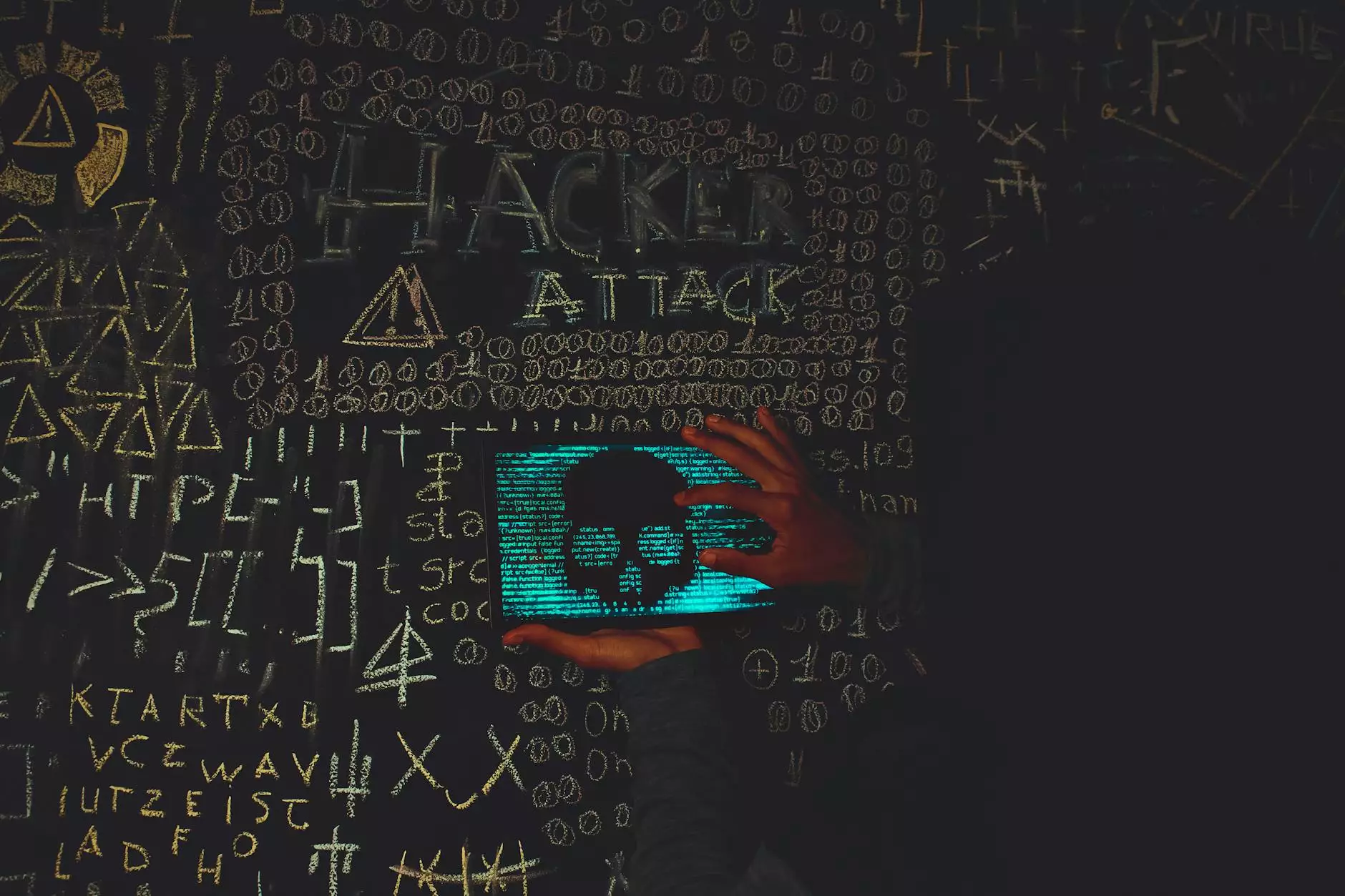
In the rapidly evolving landscape of IT services and computer repair, organizations face a daunting challenge—combatting increasingly sophisticated malware attacks. The reliance on traditional security measures often falls short. This is where malware machine learning steps onto the stage, providing a cutting-edge solution for enhanced security. This article explores the intricacies of malware machine learning and its transformative impact on security systems.
What is Malware Machine Learning?
Malware machine learning refers to the application of machine learning techniques to analyze and defend against malware. By utilizing algorithms that can learn from data, this technology identifies patterns and can effectively detect, classify, and respond to malware threats that traditional methods might miss.
Key Concepts of Malware Machine Learning
- Data Collection: Gathering extensive datasets of known malware and benign software.
- Feature Extraction: Identifying relevant features from collected data to train machine learning models.
- Model Training: Using algorithms to train models on the dataset, teaching them to recognize malware behavior.
- Prediction and Classification: Applying trained models to new software to classify it as benign or malicious.
The Importance of Machine Learning in Malware Detection
In today's digital world, threat actors are innovating at an alarming speed. Traditional signature-based detection methods struggle against zero-day attacks or newly created malware that does not have a signature. Machine learning shifts the paradigm by focusing on behaviors rather than signatures. Here are several reasons why this is essential:
1. Behavior-Based Detection
Machine learning models can identify malicious behavior based on various parameters, rather than solely relying on pre-existing signatures. This capability allows for the early detection of novel attacks, providing a crucial advantage in defending against evolving threats.
2. Real-Time Processing
The effectiveness of sandwiching real-time data processing with machine learning algorithms cannot be overstated. This empowers security systems to not only detect but also respond to potential threats instantaneously, drastically reducing the potential damage.
3. Reduced False Positives
False positives can lead to unnecessary disruptions and resource expenditures. Advanced algorithms and learning capabilities reduce the incidence of false alarms, allowing security teams to focus on genuine threats instead.
Applications of Malware Machine Learning
The versatility of malware machine learning leads to its application across various domains. Here are some significant applications:
1. Endpoint Security
In the realm of endpoint security, machine learning enhances detection capabilities on devices such as laptops, smartphones, and servers by evaluating activity patterns and identifying anomalies that may indicate a threat.
2. Network Security
On the network front, malware machine learning analyzes traffic patterns, detects irregular behavior, and blocks suspicious activities, thereby securing the entire network infrastructure.
3. Email Security
Machine learning plays a critical role in email security by analyzing incoming messages for phishing attempts and malicious attachments, thus protecting users from common entry points of attacks.
Challenges in Implementing Malware Machine Learning
While promising, implementing machine learning in cybersecurity presents certain challenges:
1. Data Quality and Quantity
The effectiveness of machine learning algorithms hinges on the quality and quantity of data. Insufficient or biased datasets can lead to inaccurate predictions and models.
2. Evolving Malware Techniques
Cybercriminals continuously evolve their tactics. As a result, machine learning models require regular updates and retraining to adapt to new methodologies used in malware development.
3. Resource Intensive
Developing and maintaining machine learning infrastructures can be resource-intensive, requiring specialized personnel and significant computational power.
The Future of Malware Machine Learning in IT Security
Looking forward, the integration of malware machine learning into IT security frameworks appears bright and holds transformative potential. Here are some trends to watch:
1. Integration with Artificial Intelligence
As machine learning progresses, its pairing with artificial intelligence will create even more potent defense systems capable of adaptive learning and proactive threat hunting.
2. Automated Response Systems
Future security solutions will likely incorporate automated response mechanisms that can isolate or neutralize threats without human intervention, thus significantly reducing response times.
3. Crowdsourced Intelligence
Crowdsourced threat intelligence platforms will provide vast datasets, enhancing machine learning models through shared knowledge of emerging threats across organizations.
Conclusion
As the digital landscape continues to evolve, understanding and utilizing malware machine learning becomes paramount for organizations aiming to safeguard their assets effectively. It is not merely a trend but a necessity in the fight against cyber threats that are ever-growing and increasingly sophisticated.
Investing in advanced security technologies such as machine learning is not just about protecting data but also about ensuring business continuity and trust among consumers. Through the fusion of machine learning and cybersecurity, organizations can achieve a sustainable competitive edge while ensuring their digital infrastructure remains resilient against potential threats.
For more insights on IT services and advanced security systems, visit spambrella.com today!