Revolutionizing Software Development: The Power of Automatic Image Annotation
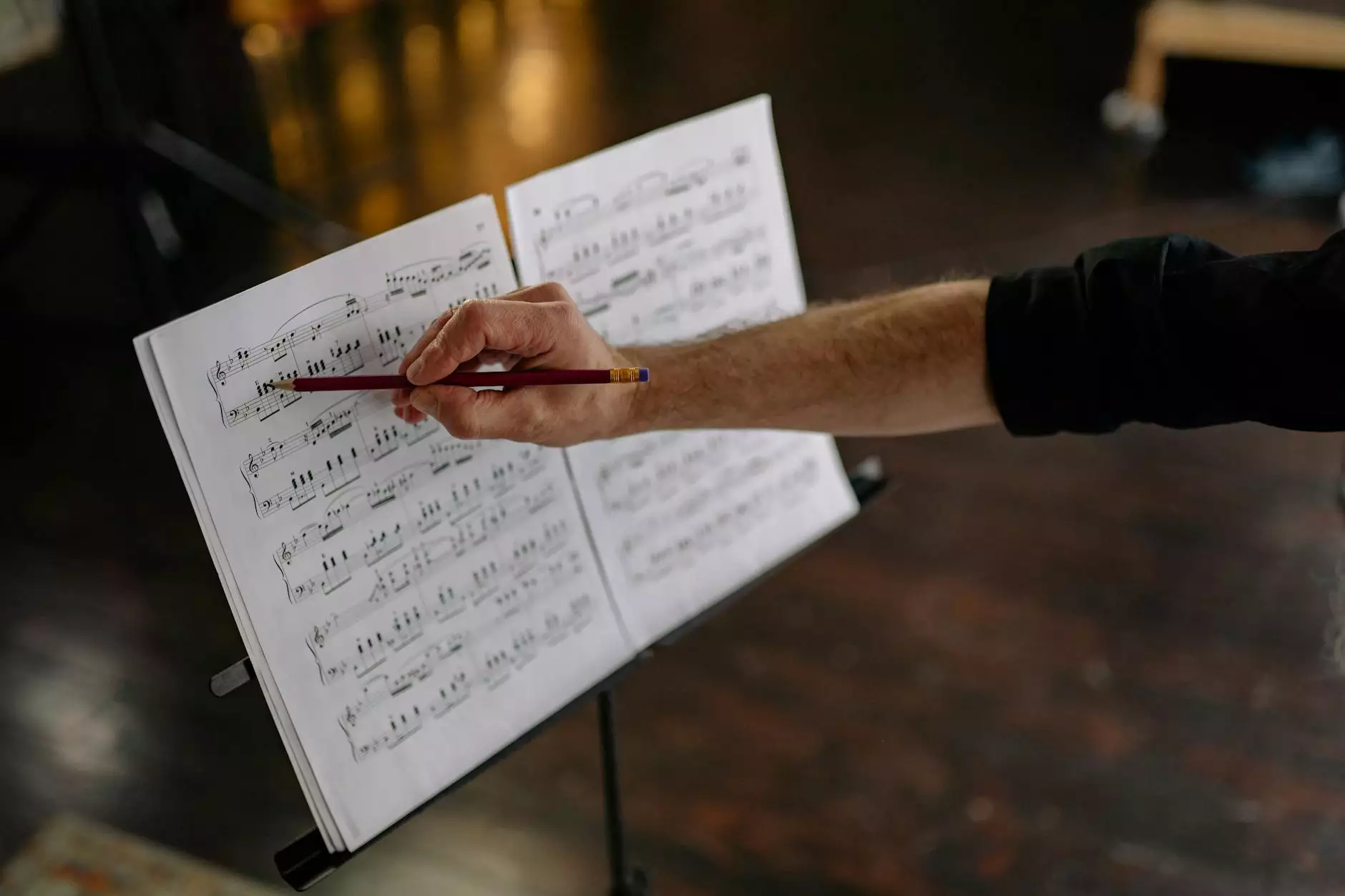
In the modern world of technological advancements, automatic image annotation emerges as a pivotal innovation that not only maximizes efficiency but also enhances the capabilities of software development. This article delves into the intricacies of automatic image annotation, exploring its profound impact on the software sector, particularly for companies like keymakr.com.
Understanding Automatic Image Annotation
At its core, automatic image annotation involves the process of automatically labeling or tagging images using various algorithms and machine learning techniques. This technology significantly reduces the time and effort traditionally spent on manual image tagging, thus paving the way for more innovative applications and solutions in software development.
How Does It Work?
The process typically involves several key steps:
- Data Collection: Large datasets of images are collected to train the algorithms.
- Machine Learning Models: Machine learning models, particularly neural networks, are trained on these datasets to recognize and label images accurately.
- Annotation Process: Once trained, the models can analyze new images and produce annotations in real-time.
- Feedback Loop: Continuous learning and adaptation through feedback improve the accuracy of annotations over time.
The Significance in Software Development
The implications of automatic image annotation stretch far and wide within the realm of software development. Here are several reasons why it is essential:
1. Enhanced Efficiency
One of the most compelling benefits of automatic image annotation is the significant increase in efficiency. Manual tagging is not only time-consuming but also prone to human error. By automating this process, developers can save countless hours that can be redirected towards more critical tasks.
2. Cost-Effectiveness
Businesses can achieve substantial cost savings by reducing the need for large teams dedicated to manual image annotation. This reallocation of resources allows for investment in other crucial areas of development, driving overall growth.
3. Improved Accuracy and Consistency
Machine learning models can provide a level of accuracy and consistency that is often unattainable through manual processes. The risk of human error is virtually eliminated, ensuring that every image is annotated with precision.
Applications of Automatic Image Annotation
The applications of automatic image annotation are diverse and span several industries. Below are a few notable examples:
1. Healthcare
In the healthcare sector, automatic image annotation is being used in medical imaging to identify conditions such as tumors in scans quickly. By tagging images with relevant information, doctors can make faster and more accurate diagnoses.
2. E-commerce
E-commerce platforms utilize automatic image annotation to enhance product searchability. By labeling product images with relevant tags, search algorithms can showcase items based on user queries more effectively, improving customer experience and sales.
3. Autonomous Vehicles
In the automotive industry, particularly with self-driving cars, automatic image annotation plays a vital role. It helps in recognizing and categorizing objects within the vehicle's environment, significantly enhancing safety and operational efficiency.
4. Social Media
Social media platforms leverage automatic image annotation to improve user interaction. Annotations can assist in tagging users in photos and suggesting related content, which keeps users engaged and increases platform activity.
Challenges in Implementing Automatic Image Annotation
Despite its numerous advantages, implementing automatic image annotation does come with challenges. Understanding these is crucial for companies looking to adopt this technology.
1. Data Quality
The effectiveness of any machine learning model, including those used for automatic image annotation, is heavily reliant on the quality of the data used for training. Poor-quality images can lead to inaccurate annotations.
2. Model Complexity
Developing advanced machine learning models can be complex and requires specialized knowledge. Companies may face difficulties finding professionals with the necessary expertise to create and implement these models effectively.
3. Continuous Learning Requirement
To maintain accuracy, models must continually learn from new data. This ongoing requirement for updating and retraining poses logistical challenges and can require significant computational resources.
The Future of Automatic Image Annotation
The future of automatic image annotation looks incredibly promising. As technology continues to evolve, we can anticipate the following trends:
1. Increased Accuracy through AI
With advancements in artificial intelligence, future models will likely provide even greater accuracy in tagging and annotating images, enabling more complex and nuanced applications.
2. Greater Integration Across Platforms
As the demand for cross-platform solutions grows, expect to see automatic image annotation integrated into more software tools, enhancing functionality and market reach for businesses like keymakr.com.
3. Broadened Use Cases
As industries continue to realize the benefits of this technology, we will likely see an expansion of its applications beyond current use cases, leading to innovative solutions that we have yet to imagine.
Conclusion
In conclusion, automatic image annotation is a transformative force in the software development landscape. Its ability to drive efficiency, enhance accuracy, and reduce costs makes it an invaluable tool for businesses striving for success in an increasingly competitive marketplace. As technology continues to progress, companies like keymakr.com are uniquely positioned to leverage the power of automatic image annotation, ensuring they remain at the forefront of innovation. The journey has just begun, and the exciting possibilities that lie ahead are limitless.